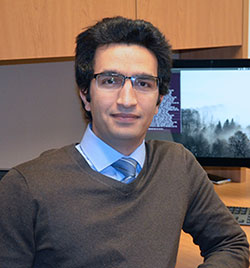
Contact Us
Institutional Communications
Bureau of Mines Building, Room 137
Laramie, WY 82071
Phone: (307) 766-2929
Email: cbaldwin@uwyo.edu
Published October 12, 2021
Two University of Wyoming researchers developed a machine learning model that improves the accuracy of detecting earthquakes by 14.5 percent compared to the most accurate current existing model.
Pejman Tahmasebi, an associate professor in the UW College of Engineering and Physical Sciences, led a study in which they have proposed a method that is more efficient in detecting seismic activity.
“Our developed model can efficiently process the signal data recorded by seismometers. It can automatically differentiate seismic events, those that are related to earthquakes and important for us, from seismic noise,” Tahmasebi says. “Moreover, when the seismic event is detected, the proposed model can provide an approximate estimation of the geographical location where it happens. The most important thing is that our model is more accurate in terms of the estimation accuracy of the geographical location, where a 14.5 percent improvement is achieved compared to the existing one.”
Tahmasebi is corresponding author of a paper titled “Attention-Based LSTM-FCN for Earthquake Detection and Location” that was published Oct. 5 in Geophysical Journal International. It is one of the world’s leading primary research journals in solid-Earth geophysics and publishes high-quality research papers on all aspects of theoretical, computational, experimental, applied and observational geophysics.
Tao Bai, a fourth-year Ph.D. student in the UW College of Engineering and Applied Science, is the paper’s lead author. Bai developed the computer codes for this research.
Earthquake detection and localization are challenging because a seismic signal is usually noisy and microearthquakes are hidden within the seismic noise, according to the paper. Traditional detection and localization methods often rely on manually chosen phases or computationally intensive algorithms.
To better differentiate seismic events from seismic noise and identify the regions in which seismic events occur, Tahmasebi and Bai developed a model that is a combination of existing long short-term memory (LSTM) and fully convolutional network (FCN) machine learning models, a combination that has not been used before. The LSTM model is used for capturing the temporal dynamics, or how a signal changes during time, of data. The FCN model is used for extracting important and hidden features of seismic events, which are difficult to extract to observe visually.
“These data actually have two components -- time and space -- since they are collected over time,” Tahmasebi says.
The model the UW researchers created has a classification accuracy of 89.1 percent, which represents a 14.5 percent improvement over the ConvNetQuake model, the state-of-the-art method, Tahmasebi says. Moreover, while the ConvNetQuake model has the capability of classifying seismic events into one of six geographic regions, the model created by the UW researchers can locate seismic events with a higher resolution by classifying seismic events in 15 regions.
“In our proposed model, the same region was divided into 15 small subregions, which means the predicted geographical location of the seismic event will be more accurate,” Tahmasebi says.
The two tested the effectiveness and efficiency of their proposed model on seismic data gathered from Oklahoma, which has experienced an increased number of earthquakes in recent years due to wastewater disposal, Tahmasebi says.
Data compiled for the study’s training set included 2,709 seismic events and 700,039 seismic noise readings, while the testing set contained 2,111 seismic events and 129,170 seismic noise readings. Although their model did not identify all seismic events (three were missed), fewer seismic noises were misclassified as seismic events, according to the study.
“The results of this study are not limited to earthquake problems,” Tahmasebi says. “The proposed method can be used to monitor volcanic activities, infrastructure modeling, clean energy, geothermal systems and other problems in which processing large data is a challenge.”
The research was funded by UW’s School of Energy Resources.
Contact Us
Institutional Communications
Bureau of Mines Building, Room 137
Laramie, WY 82071
Phone: (307) 766-2929
Email: cbaldwin@uwyo.edu